World of methodologies | Virtual control arms provide more data for decision making
Recently, there has been a lot of discussion on virtual control arms. In this blog post Data Scientist and virtual control arm specialist Johanna Vikkula explains how a virtual control arm can be formed using Real-World data (RWD) and why to use virtual control arms.
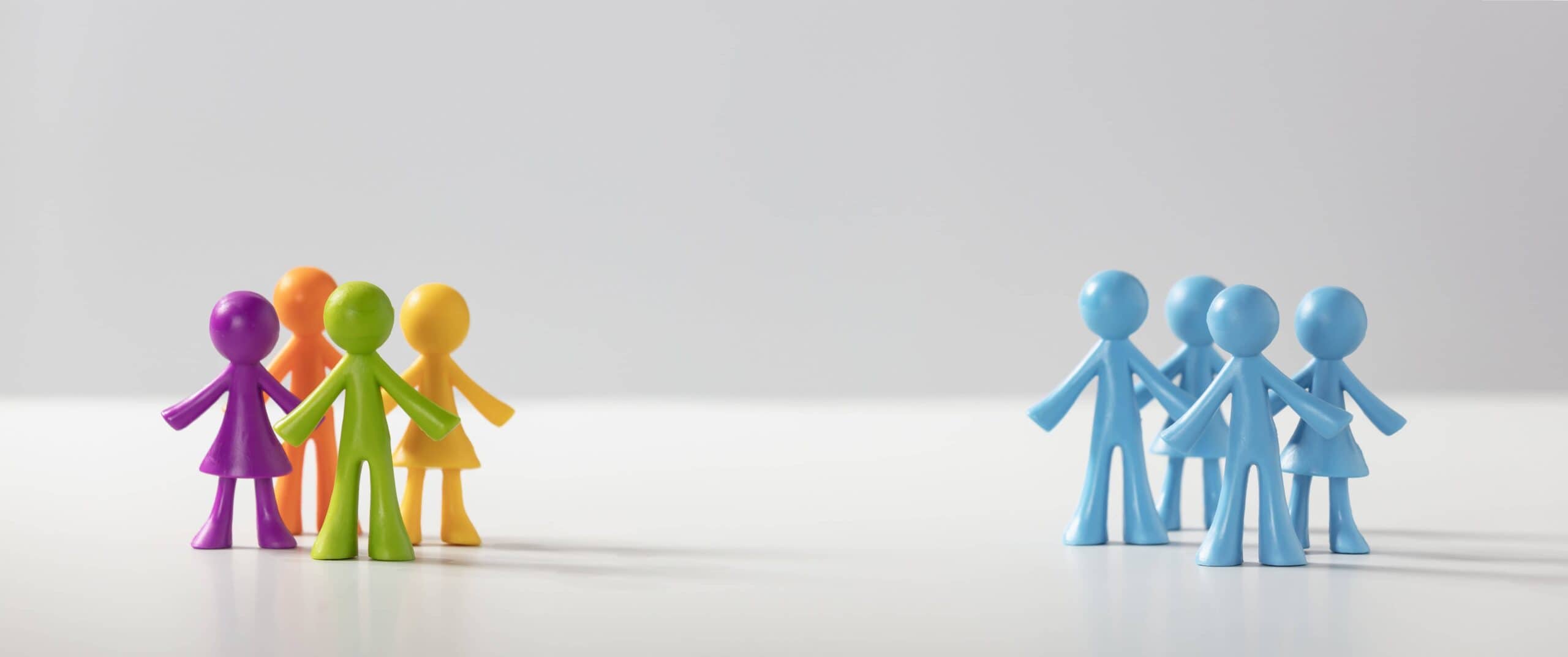
Last spring, we discussed how to complement clinical trials with RWD. This blog will dive deeper into this topic and broaden our knowledge of virtual control arms.
Virtual control arm studies are a type of control group studies – independent of clinical trials. Virtual control arms can be formed retrospectively for example by using RWD.
”Virtual control arms are utilized to complement the evidence of either single or two arm clinical trial using RWD.”
Typically, the need for a virtual control arm rises when the clinical trial is conducted as a single arm study. In these cases, the control group is not part of the trial. Therefore, the authorities may value, but also request data of a virtual control group for reimbursement and marketing authorisation purposes. In contrast, a two-arm clinical trial comprises both the subjects and the controls. Thus, virtual control arms and RWD can be used to supplement the results of the clinical trial.
Using RWD to create virtual control arms
Creation of a virtual control arm using RWD starts with similar steps as in any RWE study. Here I will describe how to create a virtual control arm step by step.
Creation of a virtual control arm
1. Data extraction
The process starts with gathering of the patient cohort. For this purpose, we can use – depending on the needs – either local data lakes or national registries. Typically, the patients are identified based on diagnosis codes.
After identifying the cohort, the data will be enriched with data of i.e. healthcare contacts, medications, and laboratory results.
After finalizing the cohort and data enrichment, we can finally start identifying the virtual control arm using the inclusion and exclusion criteria of the clinical trial. Typically, the original criteria of the clinical trial may have to be adjusted to be suitable for RWD. For example, the disease progression is rarely recorded in structured format in the registries. Therefore, disease progression is typically approximated based on treatment switches or laboratory results.
Also, some exclusion criteria of the clinical trial can be ignored. For example, some medications used for co-diagnoses may confound the clinical trial. Thus, these co-diagnoses are listed to the exclusion criteria of the clinical trial but may not be relevant when constructing the virtual control arm.
2. Patient matching
Patient matching can be performed in various ways and levels. The method is typically determined based on the availability of patient level data from the clinical trial.
Patient matching allows comparison of the results of the clinical trial and the virtual control arm. If the baseline characteristics, for example, age, co-diagnoses, and treatment history, of the virtual control arm differs notably from the clinical trial, it is impossible to recognize the source for different outcomes in the two studies. Without patient matching, we are thus skating on a thin ice in making conclusions from the virtual control arm.
Occasionally, sharing of the patient level data of the baseline characteristics from the clinical trial is not possible due to data privacy reasons. In these cases, we have to settle for using the inclusion and exclusion criteria of the clinical trial, but fancier matching cannot be performed. Therefore, some differences in baseline characteristics between the clinical trial and the virtual control study may arise and thus, direct comparison of the results may not be justified.
”Virtual control arm based on the inclusion and exclusion criteria of the clinical trial provides real world evidence of the studied cohort and is always better than nothing. However, better results can be achieved when the cohorts can be matched in regards of baseline characteristics.”
When patient level baseline characteristics data from the clinical trial can be shared, more sophisticated patient matching can be performed. This means that the virtual control arm is matched to clinical trial patients in regards of the baseline characteristics and the outcome analyses are performed using weighted models. Thus the patients similar to the clinical trial will be weighted more than the deviant ones.
In an even more sophisticated approach, all patient level data – both baseline characteristics and outcome data – of the two cohorts can be combined. This is a dream-come-true situation for patient matching and is always aimed at. However, the privacy regulations of the clinical trial may complicate or even forbid the sharing of patient level data.
3. Analyses
After the data has been extracted and matched, we can finally move forward to the analyses. Although this stage is the most interesting in terms of results, the steps before it are essential for the results to be usable.
Prospects of the virtual control arms
The quality and extent of the Finnish healthcare registries is unique. Therefore, these registries allow an excellent opportunity to perform a virtual control arm study in Finland using RWD.
In a best-case scenario, a virtual control arm may provide more data cost efficiently for authorities’ decision making. Typically, clinical trials last several years and cost millions of euros, whereas virtual control study can be conducted in a year and with a fraction of the costs.
I believe that the potential of virtual control arms is massive, even though the more sophisticated patient matching approaches require access to the patient level data of the clinical trial. In the future, the use of synthetic data for these purposes may also be possible.
We at Medaffcon have experience in virtual control arm studies and our customers’ interest seems to increase day by day. Today, virtual control arms have a huge, unharvested potential. I have a feeling that in the future, virtual control arms become more popular, and the potential will be utilized in its full capacity.